Unlocking Discriminant Analysis: Methods, Applications, and Examples
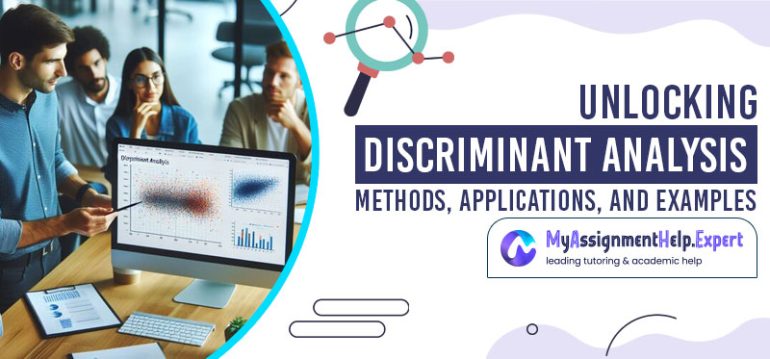
What is Discriminant Analysis?
Analysing data and extracting information from it is integral to any process, cognitive or otherwise. Mathematics, the language of nature, has ever been at the forefront of data analysis. And statistics is the branch of mathematics that purviews the methods, processes and techniques of data analysis.
Discriminant analysis is a powerful statistical method to classify and categorise multidimensional data. The process looks at the features and variables that define the data & interpreting them to discriminate the data in a set & understand the set’s intrinsic classifications & imbalances.
So, what exactly is discriminant analysis? How do we define discriminant analysis in technical terms?
Discriminant Analysis Definition
DA is a multivariate analysis technique and is heavily used in predictive/descriptive models that analyze the predictor variables of a multidimensional dataset and classify dataset elements. The metric predictors are independent and are linearly combined to ensure optimal classification. Thus, the primary goal of discriminant analysis is to find a dimension/predictor variable that can be used to design accurate classification functions.
This method finds extensive applications in data mining and supervised machine learning models as classifiers, where the predictor variables are continuous and the output needs to be categorized into discrete class labels. The class labels of the criterion or classification variable are known a priori; thus, the entire process is deemed supervised.
What are the Steps of the Discriminant Analysis Process?
The Model
The model of a DA-bases classifier generally involves a variate, linear function of multiple independent dimensions/predictor variables that can best discriminate the data involved. The variate is also known as the discriminant function, a linear combination of the most accurate predictors, each being assigned a weight to maximise the variance between dissimilar elements and reduce the variance of similar ones.
A typical discriminant function looks like this.
Every discriminant function has specific types of weights associated with them. And, every independent variable has a distinct weight associated with them, allowing for adequate classification.
The above classifier or discriminant function can involve either simultaneous or stepwise estimation. Simultaneous estimation classifies while considering all independent variables at once, while stepwise analysis considers one variable at a time based on their discriminating power.
Also, note that the above is the most basic structure of a DA function. If you are dwelling on the intricacies, here’s an excellent place to start.
So, how do we design a discriminant analysis model? What are the steps of a discriminant analysis design?
Stages of Discriminant Analysis
Step 1
The first step is problem formulation. Determine classification objectives, the predictor variables, and the category or criterion variables. Criterion variables must be mutually exclusive and exhaustive. And if they are continuous or scaled to ratios and intervals, they need to be converted into distinctive categories.
Criterion variables, dependent or categorical variables, must have specific cut-off points for every category.
Step 2
DA is a supervised learning process so will involve training data with known group memberships. The first stage of designing a model, thus, consists in acquiring appropriate training data.
If big enough, the training dataset can be divided into two groups: the estimation or analysis samples and the validation or holdout samples. Analysis samples are used to estimate the discriminant function while validation samples helped validate the classifier’s output. The two samples are often interchanged to cross-validate and improve predictions/descriptions.
Step 3
The third step is the estimation of the discriminant function weights or coefficients. The direct or simultaneous methods involve developing the discriminant function while considering all predictors, regardless of their influence or discriminating abilities.
Another alternative approach is the stepwise method. The predictors are entered one by one as per their ability to discriminate.
Certain assumptions regarding the datasets must be made, namely, normality of the predictor variables and linearity in the relationships between them, lack of multiple collinearities, and equal dispersion matrices.
Step 4
The next step is to understand whether the estimations of the discriminant functions are accurate and statistically significant. There must be an optimal cut-off score for precise classification, criteria for determining the classifier’s hit ratio, as well as the level of accuracy in its predictions.
Step 5
The discriminant classifier is then implemented, and its results are interpreted.
Step 6
The predicted results are then validated using cross-validation and noting the differences between/among the groups empirically.
Those were the critical stages in discriminant analysis.
As mentioned, discriminant analysis is a supervised classification process and is generally used when predictor variables with categorical group variables. Let’s find out where such classification processes are employed.
When To Use Discriminant Analysis?
DA is best used when multiple predictor attributes are present with only a single classification variable.
Pattern recognition, predictive analytics, data mining, business analytics and intelligence are numerous use cases of this powerful analysis technique. DA is potent enough to solve classification issues and accurately determine classification characteristics.
Numerous businesses use discriminant analysis to mine vast volumes of data and uncover trends & patterns. DA reveals potent insights that aid in decision-making, developing innovative business ideas, predicting customer behavior, & formulating robust strategies.
From predicting market trends & operational performance to evaluating human behavior, discriminant analysis finds usage across diverse statistical analysis applications.
The Objective of Discriminant Analysis with An Example
As you may have understood by now, the objective of discriminant analysis is to classify the elements of a heterogeneous dataset into distinct categories using the dimensionalities or attributes as predictors.
The following example from IBM.com can elucidate the objectives of discriminant analysis.
On average, people living in temperate regions consume more calories than tropical regions. Again, more people in temperate areas live in cities than in tropical regions. You intend to combine all such different information/attributes to design a function to discriminate a collection of countries.
Specific additional attributes also need to be considered, such as population size and economic condition.
DA allows one to use all these factors as independent predictors for distinguishing the region where a country belongs. A typical DA classifier can look like the following:
D = a * climate + b * urban + c * population + d * gross domestic product per capita + e * calorie consumption…
If the above features are the right ones for classifying the countries, then the value of D will change accordingly.
Types of Discriminant Analysis
Logically speaking, there are diverse extensions, not distinctive types of discriminant analysis. There are three essential extensions: linear discriminant analysis, quadratic discriminant analysis, and canonical or multiple discriminant analysis.
Linear Discriminant Analysis
This supervised classification approach uses independent attributes to determine the class of a dependent variable. As the name suggests, the process uses a linear combination of predictors.
Certain assumptions are made while employing this process, such as normal distribution of the independent variables, homogeneity in the variance and covariance among the attributes.
Quadratic Discriminant Analysis
A subtype of LDA, quadratic discriminant analysis, is similar to LDA. The primary difference stems from the assumption that the covariance of every category is different.
Simply put, quadratic combinations of the predictors are used to determine the category of the predicted. Except for the homogeneity of covariance, all other assumptions remain the same.
Multiple or Canonical Discriminant Analysis
This is yet another powerful dimensionality reduction technique and is closely related to principal component analysis. Given multiple interval variables (the independent predictors) and a single classification variable (the dependent predicted), canonical DA identifies the canonical variables which best classify data based on the inter-class variation.
MDA or CDA derives a linear combination of the best possible predictor variables with maximum correlation with the categories or groups involved.
And those were the primary sub-types of the discriminant analysis methods. It can be pretty challenging to master, however. Not to worry, though, as MyAssignmenthelp.expert has skilled stat experts ready to provide comprehensive aid!
How Do Our Experts Help Students In Their Discriminant Analysis & Stat Assignments?
At MyAssignmenthelp.expert, we are a 15-year-old academic service provider with a global presence and stellar reputation. Connect with our dedicated statistics assignment writing teams and get post-graduate statisticians to solve the most challenging problems quickly.
Here’s a quick overview of our most prominent features & perks.
- 5000+ Subject Matter Experts From Top Universities
- Cohesive, Domain-Specific Writing Teams
- Comprehensive Assignment Writing Assistance For Maths, Stats & More
- On-Time Deliveries
- 100% Original Solutions
- FREE Plagiarism Report
- Thorough Quality Check
- FREE blogs, guides, and online academic tools
- Pocket-Friendly Rates & Attractive Discounts
- Sign-Up, Referral, & Membership Bonuses
- 24*7 Customer Support Services
Connect with MyAssignmenthelp.expert today and get ready to defeat those devious discriminant analysis sums & stupefying statistics assignments like a pro!